Title: Neural Named Entity Recognition for Morphologically Rich Languages
Abstract:
Named entity recognition (NER) is an important task in natural language processing (NLP). Until the revival of neural network-based models for NLP, NER taggers employed traditional machine learning approaches or finite-state transducers to detect the entities in a given sentence. Neural models improved the state-of-the-art performance with sequence-based models and word embeddings. These approaches neglect the morphological information embedded in the surface forms of the words. In this thesis, we introduce two NER taggers that utilize such information, which we show to be significant for morphologically rich languages. Using these taggers, we improve the state-of-the-art performance levels for Turkish, Czech, Hungarian, Finnish, and Spanish. The ablation studies show that these improvements result from the inclusion of morphological information. We also show that it is possible for the neural network to also learn how to disambiguate morphological analyses, thereby, eliminating the dependence on external morphological disambiguators that are not always available. In the second part of this thesis, we propose a model agnostic approach for explaining any sequence-based NLP task by extending a well-known feature-attribution method. We assess the plausibility of the explanations for our NER tagger for Turkish and Finnish through several novel experiments.
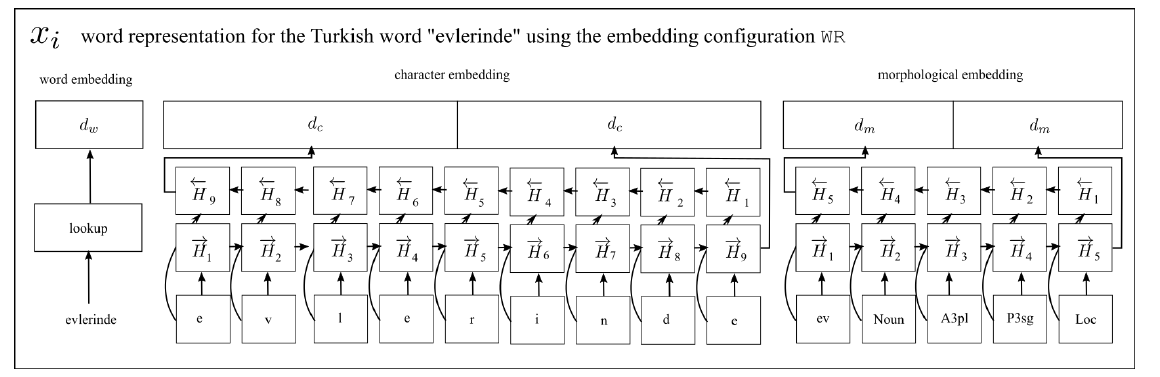